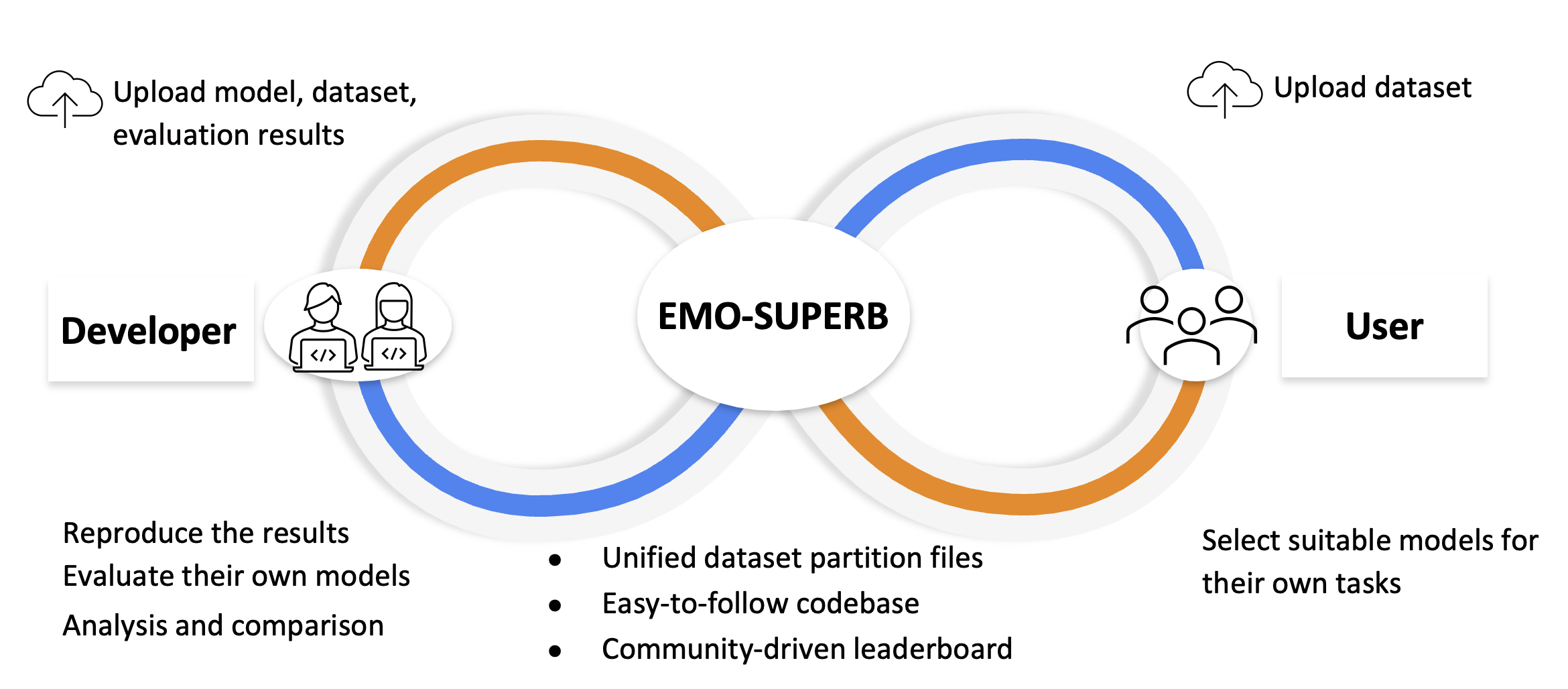
Introduction
Speech emotion recognition (SER) is a pivotal technology for human-computer interaction systems. However, 80.77% of SER papers yield results that cannot be reproduced. We develop EMO-SUPERB, shorted for EMOtion Speech Universal PERformance Benchmark, aims at enhancing open-source initiatives for SER. EMO-SUPERB includes a user-friendly codebase to leverage 15 state-of-the-art speech self-supervised learning models (SSLMs) for exhaustive evaluation across six open-source SER datasets. EMO-SUPERB streamlines result sharing via an online leaderboard, fostering collaboration within a community-driven benchmark and thereby enhancing the development of SER. On average, 2.58% annotations are annotated using natural language. SER relies on classification models and is unable to process natural languages, leading to the discarding of these valuable annotations. We prompt ChatGPT to mimic annotators, comprehend natural language annotations, and subsequently re-label the data. By utilizing labels generated by ChatGPT, we consistently achieve an average relative gain of 3.08% across all settings.